Comprehensive Guide to AI Content Moderation Types
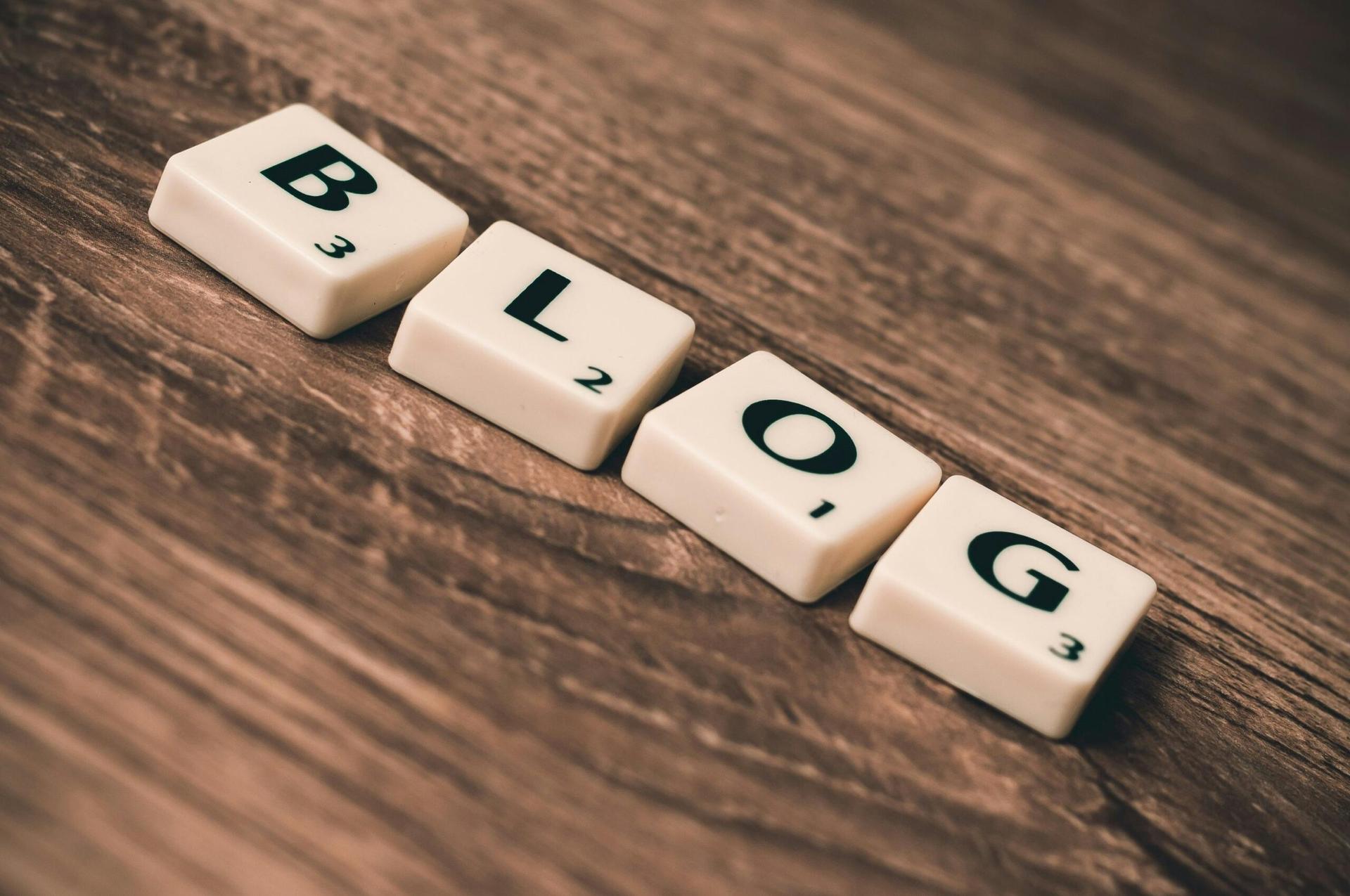
Introduction
**Can AI Content Moderation Handle the Job? Let’s Find Out!**
In today’s digital age, keeping online spaces safe is more important than ever. But can artificial intelligence (AI) step up to the challenge of content moderation? The short answer is yes, but it’s not without its hiccups. While AI can quickly scan and filter enormous amounts of digital content, sometimes it misses the finer details that are important. This is where a mix of human oversight and continuous training comes into play. In this article, we’ll delve into how AI works in content moderation, the challenges it faces, and how combining AI with human judgment can create a more secure online environment.
Can AI do content moderation?
AI can indeed perform content moderation effectively. It can quickly scan and filter vast amounts of digital content. By using machine learning algorithms, AI detects inappropriate language, hate speech, and explicit imagery. However, it isn’t flawless. Sometimes, AI struggles with context and nuances in languages. This can lead to false positives or missed harmful content. Human oversight remains essential to handle complex cases. Furthermore, continuous training and updates improve AI’s accuracy over time. With combined efforts, AI and human moderators can enhance the safety of online platforms.
How to moderate AI-generated content?
Moderating AI-generated content requires a multi-layered approach. First, establish clear guidelines for acceptable content. Use algorithms to flag potentially harmful material. Combine automated tools with human oversight for more accuracy. Train moderators to understand AI biases and limitations. Regularly update moderation rules based on feedback and new insights. Cross-check flagged content before taking action. Encourage user reporting for additional safety. Employ machine learning to continually improve moderation accuracy. Ultimately, a balanced mix of technology and human judgment ensures effective moderation. This helps maintain a safe and respectful environment.
What is automated content moderation?
Automated content moderation is the use of algorithms and artificial intelligence to monitor and manage user-generated content on digital platforms. These systems can detect and filter inappropriate, harmful, or spammy material quickly and efficiently. By leveraging machine learning, they continuously improve at recognizing nudity, hate speech, and misinformation. The primary advantage is speed and consistency in handling massive amounts of data. This technology reduces the need for human moderators, thereby cutting costs. However, concerns exist about accuracy and potential biases in the algorithms. While helpful, automated moderation isn’t perfect and often requires human oversight.
How accurate is AI in identifying harmful content?
The accuracy of AI in identifying harmful content has improved significantly over the years. Advanced algorithms and machine learning models can now detect and filter out various forms of harmful material. However, the technology is not foolproof. Sometimes, it either misses subtle harmful content or incorrectly flags benign content. Human oversight is still necessary to catch these errors. The effectiveness depends heavily on the quality of the data used to train the AI models. Continuous updates and refinements are essential. Despite its limitations, AI remains a valuable tool in managing large volumes of online content. Using it in conjunction with human moderation yields the best results.
Can AI content moderation replace human moderators entirely?
AI content moderation offers efficiency and scalability that human moderators struggle to achieve. It swiftly filters out harmful content, providing a safer online environment. However, AI lacks the nuanced understanding that humans possess. Complex issues, such as cultural context and sarcasm, often require human judgment. AI systems are also prone to biases encoded in their algorithms. Human moderators can recognize and address these biases more effectively. While AI can substantially aid the moderation process, it cannot entirely replace human moderators. The combination of AI efficiency and human insight is essential for thorough content moderation.
What role does machine learning play in AI content moderation?
Machine learning plays a crucial role in AI content moderation by enabling systems to automatically detect and filter inappropriate content. This technology uses algorithms to analyze text, images, and videos, identifying harmful material such as hate speech, violence, and explicit content. Through continuous learning, these algorithms improve over time, becoming more adept at recognizing nuanced patterns. Machine learning models can process vast amounts of data much faster than human moderators, increasing efficiency. Additionally, they aid in predicting and preventing future violations by understanding broader content trends. However, human oversight is still essential for addressing complex, context-specific issues that algorithms might miss. Overall, machine learning enhances the scalability and effectiveness of content moderation efforts.
How do AI algorithms detect hate speech and misinformation?
AI algorithms detect hate speech and misinformation using a combination of natural language processing and machine learning techniques. They analyze text for harmful patterns, flagging offensive words and phrases. These systems are trained on large datasets that include examples of hate speech and false information. Context is crucial; the AI looks at word combinations and structures, not just individual words. Advanced algorithms also evaluate user behavior and interaction history for more accurate detection. Human moderation is often involved to review flagged content and improve the algorithm over time. This multi-faceted approach helps in efficiently identifying and curbing harmful online content.
What are the challenges faced by AI in moderating multimedia content like images and videos?
AI faces numerous challenges in moderating multimedia content like images and videos. One of the primary difficulties is the complexity and variety of the content, which requires nuanced understanding. Identifying context in videos and images is often tough because AI must discern between similar-looking but contextually different scenarios. For example, distinguishing harmless nudity in art from explicit adult content can be challenging. Furthermore, AI often struggles with identifying deepfakes and other heavily manipulated media. Real-time processing adds another layer of difficulty, as it requires quick yet accurate analysis. Training these systems also demands massive amounts of labeled data, which is both time-consuming and expensive to obtain. Additionally, AI must constantly adapt to new types of content and evolving societal standards, making it a never-ending challenge.
How can AI content moderation be biased, and how can it be mitigated?
AI content moderation can be biased due to the data it’s trained on. If the training data reflects prejudiced views, those biases can be embedded in the AI’s decisions. For example, minority languages or cultural contexts might be underrepresented. This can cause misinterpretation or unfair treatment. Additionally, biases in algorithm design may favor certain groups over others. To mitigate these issues, diverse and representative training data is crucial. Regular audits and updates of the AI’s datasets can help. Including human moderators for nuanced cases also adds a layer of fairness. Collaboration with various communities can ensure a well-rounded approach.
What steps can be taken to improve the transparency of AI content moderation systems?
Improving the transparency of AI content moderation systems requires a multifaceted approach. First, companies should publicly disclose the algorithms and criteria used for moderation. This will allow users to understand how their content is evaluated. Second, regular audits by independent third parties can provide an unbiased assessment of the system’s fairness and accuracy. Third, providing clear and accessible explanations for why content is flagged or removed can help users comprehend the decisions. Moreover, offering robust channels for users to appeal against content decisions ensures fairness. Finally, engaging with a diverse group of stakeholders, including experts and community representatives, can help make the system more balanced and equitable.
How do social media platforms implement AI for content moderation?
Social media platforms implement AI for content moderation by using machine learning algorithms to detect and filter inappropriate content. These algorithms are trained on vast datasets to identify hate speech, fake news, and violent content. Advanced natural language processing helps in understanding the context of posts, while image recognition technologies scan for explicit images. When a potential violation is detected, the AI flags it for human review, ensuring accuracy. Platforms continuously update their AI models based on new types of harmful content. This combination of automated detection and human oversight aims to maintain a safe online environment.
What is the role of natural language processing (NLP) in AI content moderation?
Natural language processing (NLP) plays a crucial role in AI content moderation. It enables machines to understand, interpret, and respond to human language. NLP algorithms can identify inappropriate or harmful content by analyzing text for offensive words, harmful phrases, and contextual cues. They can automate the process of filtering out unwanted content, ensuring that online platforms remain safe and welcoming. Additionally, NLP can detect subtleties like sarcasm or double meanings, which helps in more accurate moderation. Human moderators can then review the flagged content more efficiently. This blend of automation and human oversight makes content moderation more effective.
How do different cultural and linguistic contexts impact AI content moderation?
Different cultural and linguistic contexts significantly impact AI content moderation. AI systems often struggle with nuances and idiomatic expressions. They may misinterpret slang or context-specific language unique to certain cultures. Moderation tools trained predominantly on English data may falter with non-English content. Cultural norms also vary widely; what is acceptable in one culture might be offensive in another. For instance, symbols or gestures can have vastly different meanings across societies. Therefore, a one-size-fits-all approach in AI moderation is ineffective. Customization is crucial for achieving accuracy and fairness. Local linguistic and cultural expertise should be integrated into AI algorithms. This ensures sensitivity and appropriateness in diverse contexts.
What are the privacy concerns related to AI content moderation?
Privacy concerns related to AI content moderation are significant. One major issue is data collection. AI systems often require large amounts of personal data to function effectively. This data can include sensitive information. Unauthorized access to such data poses a risk of privacy breaches. Additionally, AI algorithms can sometimes misinterpret context. This can lead to wrongful flagging or suppression of legitimate content. Users may also be unaware of how their data is being used. Lack of transparency exacerbates mistrust among the public. Finally, centralized data storage can be a target for cyberattacks, further endangering user privacy.
How is the effectiveness of AI content moderation measured and evaluated?
The effectiveness of AI content moderation is measured and evaluated through several key metrics. Accuracy is paramount, gauging how well the AI can correctly identify and flag inappropriate content. Precision and recall are also critical, with precision measuring the percentage of true positive identifications out of all identified instances, and recall assessing the percentage of true positives detected out of all actual instances present. User feedback provides additional insights, highlighting any false positives or negatives that may occur. Timeliness is evaluated, noting how quickly the AI can process and act on flagged content. Regular audits and human reviews act as benchmarks, comparing AI decisions against human judgments. This combination of quantitative and qualitative measures ensures a comprehensive evaluation of the system’s performance.
What are some of the most advanced AI tools currently used for content moderation?
Some of the most advanced AI tools currently used for content moderation include OpenAI’s GPT-4 and Google’s BERT. These models excel at understanding and generating human-like text, which helps in identifying inappropriate content. Facebook’s DeepText leverages advanced natural language processing to interpret nuanced meanings. Another powerful tool, Jigsaw’s Perspective API, uses machine learning to detect toxic language, enhancing the moderation process. Companies also employ Microsoft’s Azure Content Moderator, which combines multiple machine learning models to scan text and images. These tools significantly enhance the ability to manage and filter large volumes of user-generated content effectively.
Blog Letters on Brown Wood. Credit: Pixabay, via Pexels.
Summary
The article explores AI’s role in content moderation, highlighting its abilities and challenges. AI can quickly scan and filter vast amounts of digital content using machine learning algorithms, detecting inappropriate language, hate speech, and explicit imagery. However, AI struggles with context and nuances, requiring human oversight for complex cases. Continuous training improves AI accuracy over time. A balanced approach combining AI with human judgment is essential to enhance online safety and fairness. Bias in AI moderation can be mitigated through diverse training data and regular audits. Transparency is crucial, with companies disclosing moderation criteria and providing clear explanations. Human and AI collaboration is necessary for effective content moderation in different cultural and linguistic contexts.